RESOURCES
Leveraging Marketing Mix Modeling for Effective Channel Mix
Today, advertisers have access to an abundance of marketing channels — both traditional channels like television and radio, as well as evolving digital channels like social media platforms, search engines, video and streaming services, and e-commerce marketplaces. However, this abundance of channels can create a challenge for advertisers who are looking to make the most impact with a tight budget, or are just unsure of what the ideal channel mix is for their goals. And of course, there’s always wanting to measure the impact.
To help drive effective strategy and make the best recommendations for clients, Code3 leverages Robyn, an open-source MMM (Marketing Mix Modeling) library created by Meta’s Marketing Science division, to conduct Marketing Mix Modeling analysis. Robyn analyzes historical campaign data using AI and machine learning statistical methods to build models which can help advertisers identify their brand’s optimal channel mix.
Keep reading for an overview of how Robyn works, and how to interpret its outputs to enable actionable and meaningful outcomes for your brand.
What to Know About Media Mix Modeling and Robyn
Media Mix Modeling is a statistical technique that has been around for decades. It uses multi-linear regression to determine the relationship between media variables, such as ad spend across channels, and the output variables, such as sales or conversions.
Robyn MMM modeling takes into account both carryover (ad stock effect) and saturation effects (diminishing returns), aspects often overlooked in traditional attribution models. By doing so, Robyn assesses the long-term impact of historical ads, providing marketers with high-level insight into their specific marketing tactics over a long period of time.
Ad Stock Effect
The carryover or ad stock effect describes the impact of advertising influencing consumer behavior after exposure to an ad. For example, a user sees an ad today and decides to make a purchase a week later. Ad stock effect can measure purchases and other business outcome metrics like revenue and registrations.
Ad stock is important because it acknowledges the impact of advertising extends beyond the moment of exposure. It recognizes that consumers might not immediately act upon seeing an ad, but may be influenced by it over time.
Ad stock has a decaying curve graph representing the effectiveness of an ad diminishing over time. It is like throwing a stone into a pond: the initial splash (the first exposure to an ad) is noticeable, but as time passes, the ripples (the influence of the ad) gradually fade. Ad stock helps marketers understand how long these ripples last and how effective the impact of an ad remains over time.
In Robyn, we can choose between 3 types of ad stock: Geometric (fixed rate), Weibull CDF (flexible rate), Weibull PDF (same as CDF, but offers lagged effect). In the below example, we modeled ad stock using Weibull CDF:
The Importance of Half-Life Points
When reading ad stock graphs it is important to see where half-life points lie. Half-life points refers to the duration it takes for the impact or effectiveness of an advertisement to decrease by half. For example, if an ad has a half-life of two weeks, it means that after two weeks, the effect of the initial exposure to the ad has diminished by half. While certain academic studies propose a half-life range of approximately 7–12 weeks, industry practitioners commonly indicate half-lives falling within the 2–5 week range.
The Importance of Diminishing Returns
Diminishing returns (also called saturation effects) is an important metric to pay attention to. It accounts for the fact that the relationship between the amount of money invested in a particular channel and the additional sales revenue or conversions generated is neither straightforward nor linear. Instead, at some level it reaches a point of saturation (inflection point) where the return per dollar spent begins to decrease:
Initially, as ad spend increases there is a rapid rise in sales revenue, user engagement, and conversions. However, as more budget is allocated to advertisements, the incremental gains in revenue, engagement, and conversions become less significant. This is because the available audience on the platform can become saturated with your ads, limiting how much more impact additional spend on the channel will have.
Understanding diminishing returns is critical, because it helps marketers understand which channels are worth incremental investment and allows them to make informed decisions about where to allocate those dollars.
Robyn leverages S-curve graphs to model this effect and calculates the diminishing return curve for each channel for which data is provided. The example below includes S-curves for Facebook, Instagram, Reddit, and Snapchat data:
The Code3 Robyn Case Studies
In our case studies, Code3 examined three budget allocation scenarios to determine the optimal budget mix that maximizes revenue. However, it is also possible to use different target variables like link clicks, revenue, or registrations, depending on the available input data and desired model outcome.
The initial scenario (represented in gray) maintains the same channel mix spending levels as the last two weeks of provided input data. It predicts that without any changes in channel mix budgets, the ROAS will be 1.64x.
The second scenario (represented in blue) allows budgets to shift up to 25% between channels. This scenario predicts a 7% increase in revenue and a ROAS of 1.75x.
The third scenario (represented in brown) allows budgets to shift up to 50% between channels. This scenario predicts a 16% increase in revenue and a ROAS of 1.89x.
Marginal Return on Ad Spend (mROAS)
While ROAS describes the ratio of past revenue vs past ad spend and is a typical metric used in performance and budget analysis, Robyn focuses on future performance by using Marginal Return on Ad Spend (mROAS). mROAS helps predict whether ROAS will increase or decrease for the next dollar spent.
When Code3 Strategists look at different channels where brands can advertise, we want to know which platform will give us the most revenue for every extra dollar we spend. Robyn gives us a clear direction on where we can get the most extra revenue for additional dollars spent.
Code3's Capabilities
Code3 recommends leveraging Robyn’s MMM modeling capability when your brand utilizes a complex mix of marketing channels and wants to understand the incremental contribution available to each channel. Don’t be afraid to utilize Robyn’s outputs and data to update your general strategy, or even to guide an incremental testing budget.
Robyn budget allocation scenarios can consider not only on platform but also by additional dimensions like funnel stage, campaign objective, or audience type, making it incredibly valuable for brands address crucial budget allocation challenges like:
- Assessing the per-channel impact of existing channel mix
- Strategically allocating media budgets to achieve the optimal marketing mix
- Understanding revenue forecasts across different budget allocation scenarios
Ready to learn more? Contact us to discover how we can assist you in a detailed review of Robyn marketing mix modeling and explore its application for your brands.
SIMILAR POSTS YOU MIGHT BE INTERESTED IN:
SIGN UP FOR OUR WEEKLY NEWSLETTER
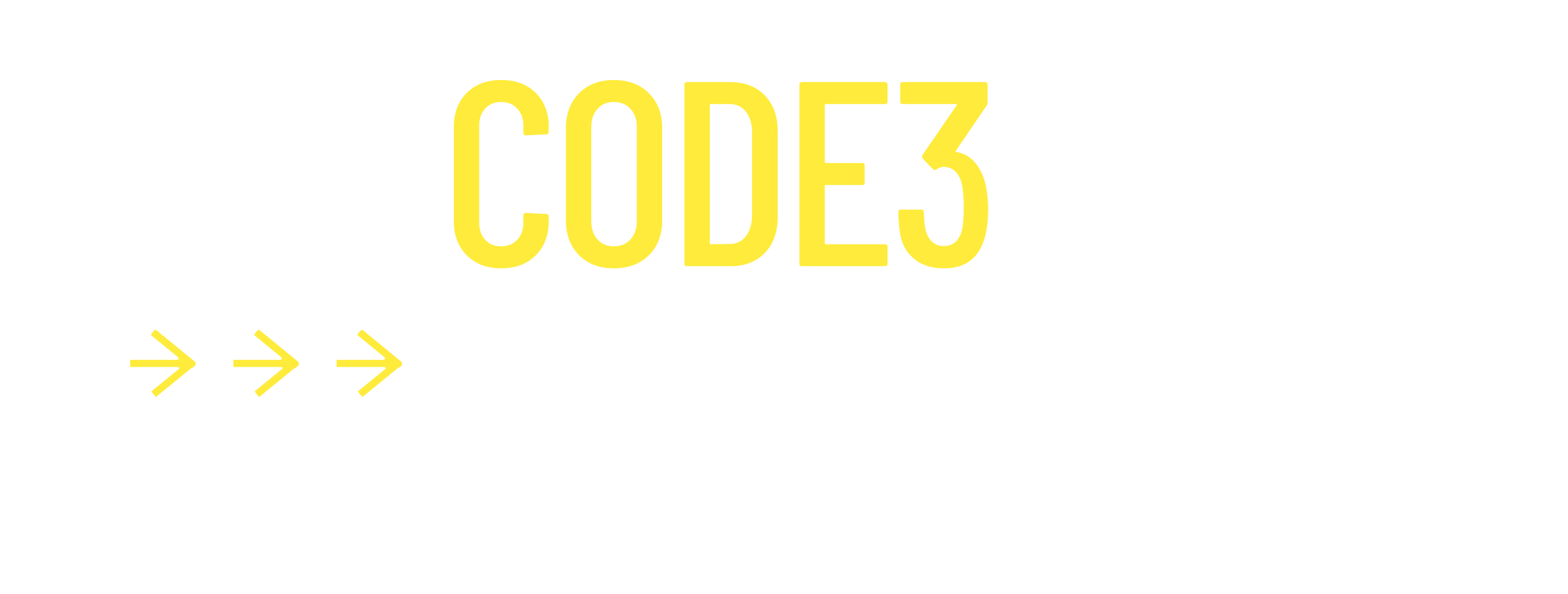
News, Views, and Valuable Resources
Delivered to Your Inbox Each Week